Introduction
Navigating the ethical landscape of AI is becoming increasingly crucial as artificial intelligence integrates into various aspects of our lives. privacy concerns, and accountability issues. Additionally, it explores practical solutions and frameworks being developed to ensure responsible AI deployment. Join us as we uncover the complexities of ethical AI and provide actionable insights for businesses, developers, and policymakers to navigate this evolving field responsibly.
- Introduction
- Section 1: Understanding the Ethical Issues in AI
- Section 2: Key Ethical Challenges in AI
- Section 3: Potential Solutions and Best Practices
- Section 4: Frameworks and Guidelines for Ethical AI
- Section 5: Case Studies and Real-World Examples
- FAQ: Navigating the Ethical Landscape of AI: Challenges and Solutions
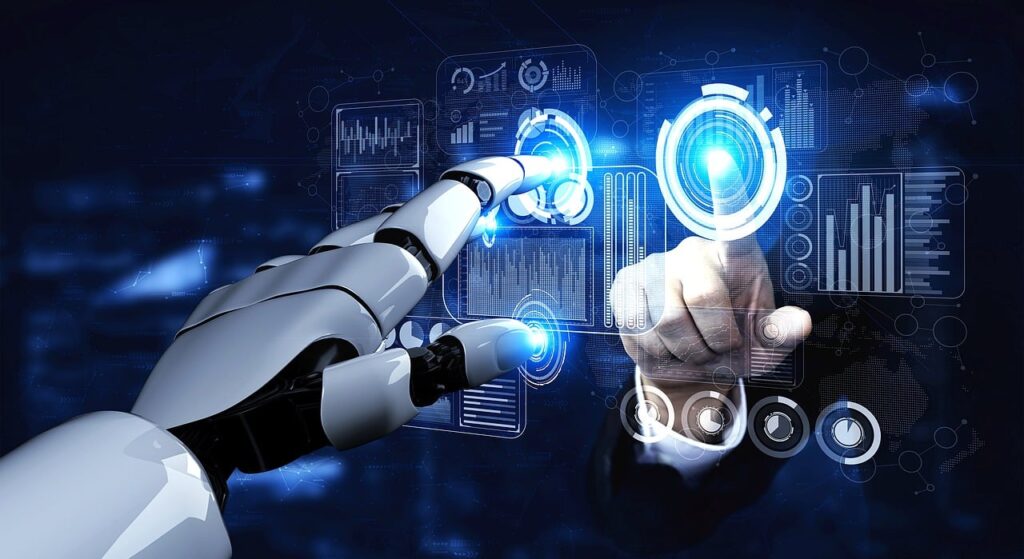
Section 1: Understanding the Ethical Issues in AI
Definition of Ethical AI: Ethical AI refers to the design, development, and deployment of AI systems in ways that are aligned with moral principles and societal values.
Importance of Ethics in AI: Ensuring AI systems operate fairly, transparently, and responsibly is crucial to building trust and preventing harm.
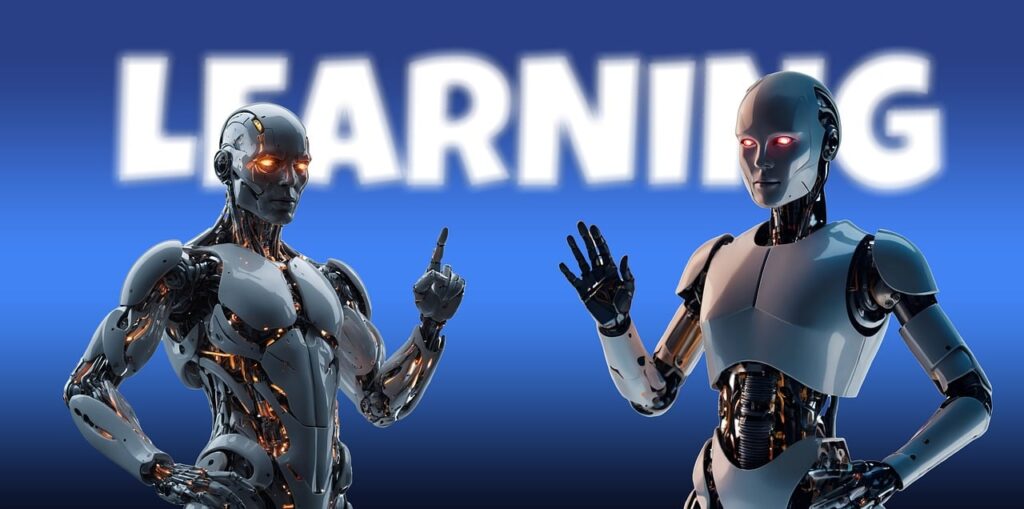
Section 2: Key Ethical Challenges in AI
Bias and Fairness
Examples: AI systems used in hiring processes or law enforcement that reflect societal biases.
Causes: Training data that lacks diversity or reflects existing prejudices.
Privacy and Data Security
Concerns: Unauthorized data collection and breaches.
Examples: Incidents where personal data was exposed or misused.
Transparency and Explainability
Importance: Users need to understand AI decision-making processes.
Challenges: Complex models like neural networks are often ‘black boxes.’
Accountability
Responsibility: Determining who is accountable for AI decisions.
Challenges: Legal frameworks lagging behind technological advancements.
Autonomy and Control
Risks: Autonomous AI systems making critical decisions without human oversight.
Examples: Self-driving cars and autonomous weapons.
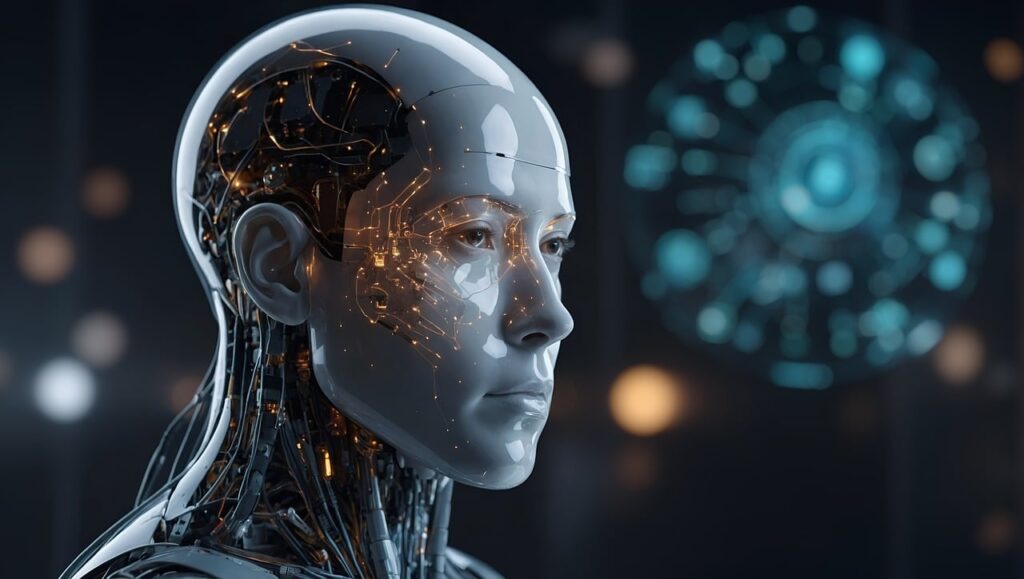
Section 3: Potential Solutions and Best Practices
Bias Mitigation Techniques
Strategies: Diverse training datasets, fairness-aware algorithms.
Research: Ongoing studies to improve bias detection and reduction.
Enhancing Privacy and Security
Techniques: Data anonymization, secure data handling practices.
Frameworks: Implementing data governance policies.
Improving Transparency and Explainability
Tools: Interpretable models, visualization tools.
Education: Informing users about AI decision-making processes.
Establishing Accountability Frameworks
Guidelines: Creating responsibility matrices and ethical guidelines.
Examples: Regulatory frameworks like GDPR.
Ensuring Human Oversight and Control
Practices: Human-in-the-loop systems, fail-safes.
Design: Mechanisms for human override.
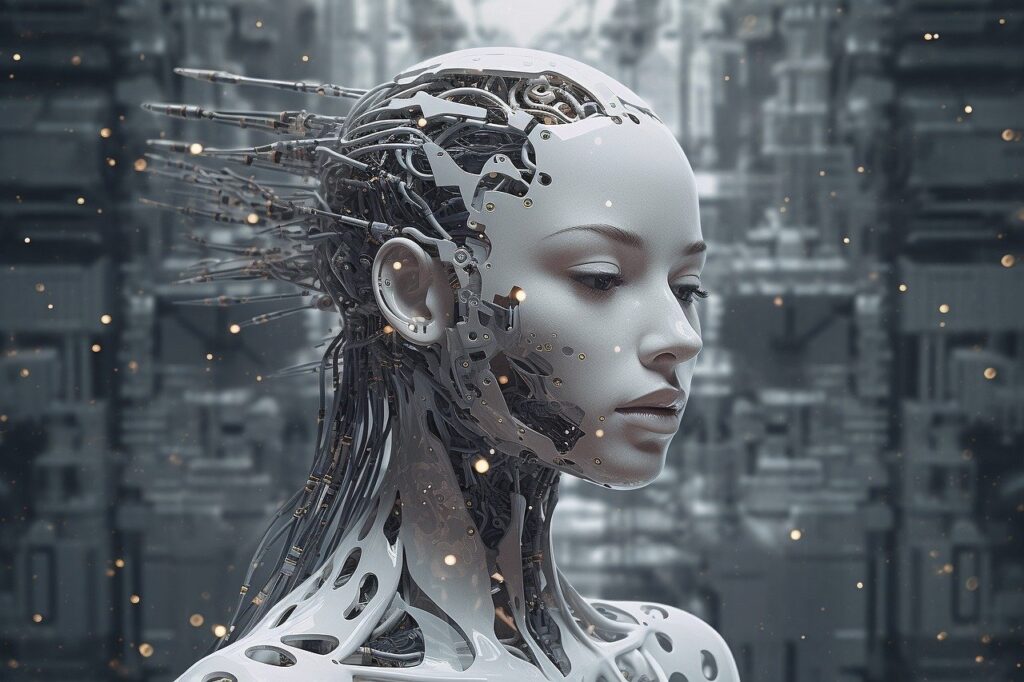
Section 4: Frameworks and Guidelines for Ethical AI
International and National Regulations
Overview: Key regulations such as GDPR and the AI Act.
Impact: How these regulations influence AI practices.
Industry Standards and Best Practices
Standards: IEEE, ISO guidelines.
Best Practices: Leading examples from industry leaders.
Ethical AI Frameworks
Frameworks: AI4People, IBM’s AI Ethics Board.
Implementation: Practical steps for adopting these frameworks.
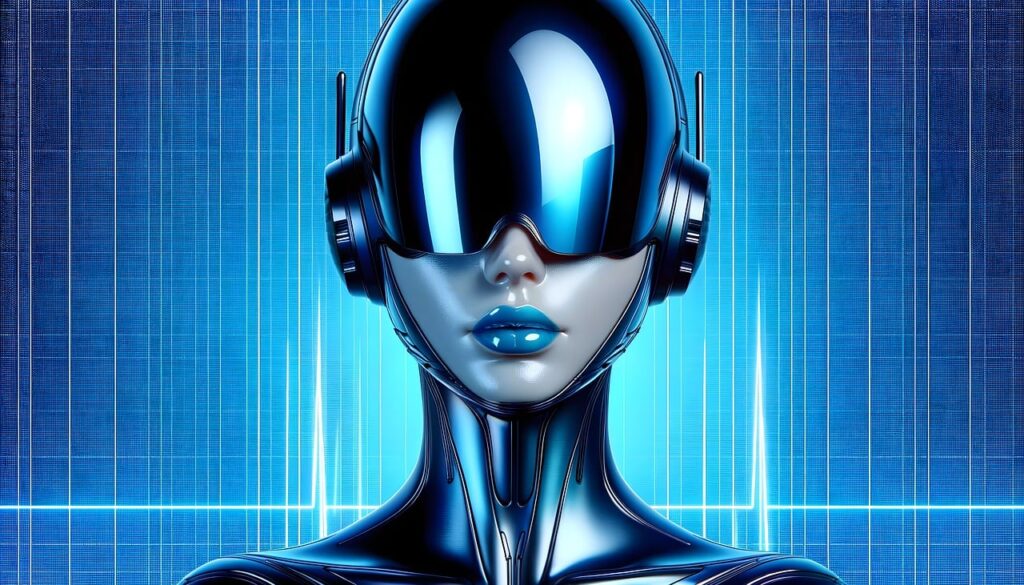
Section 5: Case Studies and Real-World Examples
Implementations: Successful examples of ethical AI practices.
Lessons Learned: Insights from organizations that faced and addressed ethical challenges.
Conclusion
In summary, navigating the ethical landscape of AI is essential as AI becomes more integrated into our lives. Addressing issues like bias, privacy, transparency, accountability, and control is crucial for responsible AI deployment. By implementing diverse datasets, robust security frameworks, interpretable models, and clear accountability measures, we can build trustworthy AI systems. Adhering to regulations and industry standards, and learning from real-world case studies, provides a practical path forward. Ensuring ethical AI development will help maximize its benefits while minimizing potential harms, fostering a positive impact on society.