Introduction
Explore the future of brain-inspired tech with neuromorphic computing efficiency, adaptability, and real-time processing advantages.
Recent advancements in technology have brought us closer to creating truly intelligent machines, but significant challenges remain. Traditional computer architectures, while powerful, often struggle with tasks requiring learning from minimal data, energy efficiency, and real-time adaptation. This is where neuromorphic computing comes into play, offering a promising avenue for creating systems that mimic the efficiency and adaptability of the human brain.
What is Neuromorphic Computing?
Neuromorphic computing designs computer systems inspired by the structure and functioning of the human brain. Unlike conventional computers, which rely on the von Neumann architecture with separate units for processing and memory, neuromorphic systems integrate these elements more closely, similar to neurons and synapses in the brain. This approach enables more efficient data processing and energy consumption.
Key Components of Neuromorphic Systems
Neurons and Synapses
In neuromorphic hardware, artificial neurons and synapses replicate the communication process in the brain. These elements can be implemented using specialized circuits or advanced materials like memristors, which change their resistance based on historical input.
Spiking Neural Networks (SNNs)
Unlike traditional neural networks that process information continuously, SNNs transmit data through discrete spikes of activity, much like biological neurons. This makes SNNs more efficient and capable of processing real-time data.
Plasticity
One of the hallmarks of the human brain is its ability to adapt and reorganize itself. Neuromorphic systems aim to incorporate plasticity, allowing them to learn and adapt over time without extensive reprogramming.
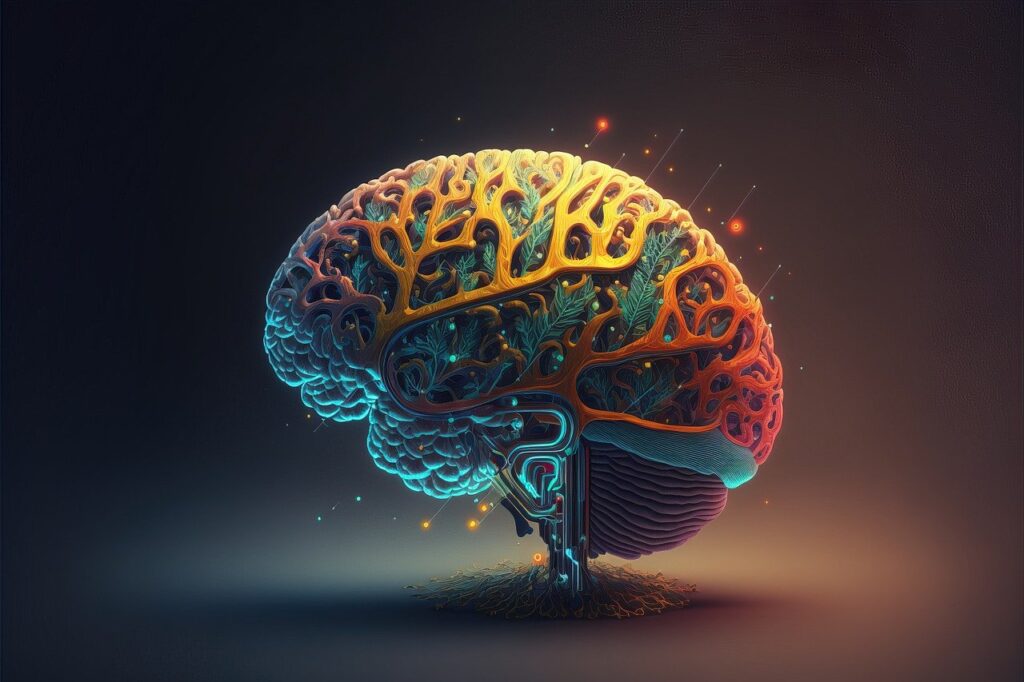
Advantages of Neuromorphic Computing
Energy Efficiency
Traditional models, especially deep learning networks, require significant computational power and energy. Neuromorphic systems, by emulating the brain’s efficiency, can perform complex tasks with much lower energy consumption.
Real-Time Processing
The brain’s ability to process information in real-time is a key advantage. Neuromorphic systems can handle dynamic data streams and make decisions rapidly, making them suitable for applications like autonomous vehicles, robotics, and real-time data analytics.
Strength and flexibility
Neuromorphic architectures are inherently more robust and adaptable. They can continue to function even when parts of the system fail, and they can learn and adapt to new situations without requiring extensive retraining.
Applications of Neuromorphic Computing
Healthcare
Neuromorphic computing can revolutionize healthcare by enabling more efficient and adaptive medical devices. For instance, brain-machine interfaces (BMIs) could become more responsive and intuitive, aiding in the treatment of neurological disorders.
Autonomous Systems
Autonomous vehicles and drones can benefit from the real-time processing capabilities of neuromorphic systems, improving their ability to navigate complex environments and make split-second decisions.
Edge Computing
In edge computing, where processing power is limited, neuromorphic systems can provide powerful capabilities without the need for constant cloud connectivity, enhancing privacy and reducing latency.
Challenges and Future Directions
While neuromorphic computing holds great promise, several challenges remain. Developing efficient and scalable hardware that can truly replicate the brain’s complexity is a significant hurdle. Moreover, creating software and algorithms that can fully exploit the potential of neuromorphic hardware requires further research and innovation. Future advancements may involve hybrid systems that combine traditional and neuromorphic computing, leveraging the strengths of both approaches. Additionally, interdisciplinary collaboration between neuroscientists, engineers, and computer scientists will be crucial in driving this field forward.
Conclusion
Neuromorphic computing represents a significant leap toward more intelligent, efficient, and adaptable systems. By drawing inspiration from the brain, these technologies have the potential to overcome many of the limitations of current architectures. As research and development in this field continue to advance, neuromorphic computing could play a pivotal role in the future of technology, bringing us closer to truly brain-inspired systems.